Like other major industries, the aftermarket industry is poised for significant change due to the sudden and rapid introduction of Artificial Intelligence (AI).
According to IBM, 34% of companies currently use AI, and an additional 42% are exploring AI. Plus, 35% of organizations are training and reskilling their teams to use new AI and automation tools to make their daily workload more efficient. While AI programs like Sora or Chat-GPT capture headlines with on-demand video production or fake images that look unnervingly like the real thing, the true impact of AI will be seen in how businesses get work done.
A recent Accenture report forecasts that the manufacturing sector will see the most significant gains from AI adoption, with gains of $3.8 trillion expected by 2035. Given this information, it is no surprise that AI has the potential to alter the aftermarket sectors’ economic impact in significant ways.
Let’s explore how AI will affect the aftermarket business in the coming years.
AI-Driven Market Analysis
Gartner Research indicates that as of July 2023, 79% of corporate strategists said technology like artificial intelligence would be critical to their success in the next two years.
Connecting with customers and predicting the market has always been one of the biggest challenges for aftermarket companies. In the past, companies needed to combine automated and manual tasks to try and determine which direction an aftermarket business wanted to go. Combining data from different sources, such as sales figures, surveys, customer feedback, and external market data, was essential for a comprehensive market analysis.
Integrating and analyzing these data sources was often challenging and time-consuming due to the lack of advanced analytical tools. AI fundamentally changes how aftermarket companies determine market direction.
How AI Algorithms Analyze Market Trends and Consumer Behavior
Advanced AI algorithms have revolutionized companies’ analysis of market trends and consumer behavior, particularly in the automotive aftermarket industry. These algorithms leverage vast amounts of data to uncover patterns, predict future trends, and provide actionable insights that can significantly impact business strategies.
Here’s how AI gets it done:
Data Aggregation: AI algorithms begin by aggregating data from various sources, including sales records, online browsing behavior, social media, customer reviews, and external market reports. This comprehensive data collection is foundational to understanding market dynamics and consumer preferences.
Pattern Recognition: Through machine learning, AI algorithms can identify patterns and trends within the aggregated data. For instance, they might detect an increasing interest in electric vehicle parts based on search trends and social media discussions. This ability to recognize patterns enables businesses to understand current market conditions and anticipate changes.
Predictive Analysis: AI algorithms use identified patterns to forecast future market trends and consumer behavior. For example, they can predict which aftermarket parts will likely see increased demand based on trends in vehicle sales, emerging automotive technologies, or seasonal variations.
Personalization and Segmentation: AI algorithms analyze consumer behavior to segment customers into groups with similar preferences or buying habits. This enables companies to tailor marketing efforts and product offerings to meet each segment’s needs better, enhancing customer satisfaction and loyalty.
Sentiment Analysis: AI can gauge public sentiment towards certain products or brands by analyzing customer reviews and social media posts. This insight helps companies address potential issues early and adapt their strategies to meet consumer expectations.
Enhanced Customer Experience
According to Hubspot, a staggering 93% of customers are likely to make repeat purchases with companies that offer excellent customer service.
Excellent customer service is the cornerstone of any business, but this is especially true in the automotive aftermarket space. Accurate product fitment information, installation support, and warranty claims are just a few areas where buyers expect aftermarket manufacturers to exceed expectations.
AI chatbots are one of the most obvious ways for companies in the automotive aftermarket space to improve their customer service experience efficiently. These automated assistants provide instant support with immediate responses to customer inquiries, reducing wait times and improving customer satisfaction. Chatbots can handle thousands of interactions simultaneously, making them cost-effective for businesses of all sizes. Plus, chatbots learn from each interaction, enabling personalized responses that improve the customer experience over time.
ACES and PIES Data
Managing ACES and PIES fitment data is essential to running a successful aftermarket business.
Since AI needs context or existing information, new products or product lines require a person(s) to ensure the correct fitment data is uploaded to each product listing. To help automate the process beyond initial creation, companies like PDM offer PIM (product information management) software to help make fitment data work across all your eCommerce platforms. This helps increase efficiencies across multiple levels of your organization and ensures a fantastic customer experience thanks to accurate fitment information.
Accurate ACES and PIES data is also fundamental to reducing returns by providing the correct information received in the first place.
Supply Chain Optimization
According to a survey conducted by Gartner, Inc., leading supply chain organizations are adopting artificial intelligence and machine learning (AI/ML) technologies to enhance their operations at a rate more than double that of their lower-performing counterparts.
By harnessing the power of AI, companies can achieve a level of precision in inventory control that significantly reduces waste, optimizes stock levels, and ensures products are available when and where they are needed.
Let’s dig in more.
Predictive Analysis for Stock Levels
Algorithms analyze historical sales data, seasonal trends, market conditions, and even socio-economic indicators to predict future product demand accurately. This predictive capability allows companies to maintain optimal stock levels, reducing overstock and stockouts.
In addition, AI systems can adjust predictions based on real-time data, such as sudden changes in demand or supply chain disruptions. This agility ensures that inventory levels remain aligned with current market conditions.
Automated Reorder Processes
Advanced AI dynamically calculates reorder points for each product based on its sales velocity, lead times, and the company’s desired service level. This automated process ensures that replenishment orders are triggered optimally to maintain stock availability without tying up excessive capital in inventory. By analyzing supplier reliability, quality, and lead times, AI can recommend the best suppliers for reorder processes, optimizing the supply chain’s efficiency and responsiveness.
Enhanced Inventory Allocation and Distribution
AI-powered tools analyze sales data across different locations to identify patterns, enabling businesses to allocate inventory more effectively to meet regional demand variations. Plus, AI can suggest the most efficient distribution strategies, considering factors like transportation costs, warehouse capacities, and delivery times. This optimization ensures that inventory is available and positioned to minimize logistics costs.
Reduction of Waste and Overstock
By providing accurate demand forecasts, AI significantly reduces the risk of overstock, which can tie up capital and result in discounted sales to clear excess inventory.
Continuous Learning and Improvement
AI systems continually learn from new data, improving their accuracy over time. This learning process means inventory management becomes more efficient as the system gains more insights into demand patterns and supply chain dynamics. To help refine results, AI can incorporate feedback from inventory performance (e.g., instances of stockouts or overstock) to refine its algorithms, ensuring continuous improvement in inventory management processes.
Integration with Other Systems
AI systems often integrate seamlessly with existing Enterprise Resource Planning (ERP) and Supply Chain Management (SCM) systems, allowing for a holistic inventory and order management approach across the supply chain.
Section 4: AI in Manufacturing and Quality Control
Machine Learning and AI significantly improve quality control in product manufacturing by examining extensive datasets to discern patterns and irregularities. This enhances the ability to detect defects, foresee potential problems, and refine manufacturing workflows. Additionally, these technologies help minimize human error, thereby boosting the overall effectiveness of quality assurance practices.
Check out this real-world example of AI in action from the aftermarket components experts at Bilstein.
Automated Visual Inspection Systems
Bilstein implemented an AI-powered visual inspection system to identify defects in automotive components. The system uses high-resolution cameras to capture images of each part moving along the production line.
Machine Learning Model
These images are analyzed by a machine learning model trained to recognize defects such as cracks, scratches, or incorrect dimensions. The model was trained on a dataset comprising thousands of images of defective and non-defective parts, learning to differentiate between the two with high precision.
Real-time Quality Control
When the system detects a potential defect, it automatically flags the part for further inspection or removal from the production line. This real-time quality control process significantly reduces the rate of defective parts reaching customers, enhancing product quality and customer satisfaction.
Continuous Improvement
The machine learning model continuously improves over time as it is exposed to more data, increasing its accuracy and reliability in defect detection.
This example showcases how AI technologies like machine learning and computer vision revolutionize quality control in aftermarket part manufacturing, ensuring high product quality standards while optimizing production efficiency.
Challenges and Considerations
65% of consumers say they’ll still trust businesses who use AI – Forbes Advisor
Integrating artificial intelligence (AI) into existing systems in the automotive aftermarket and other industries presents challenges that companies must navigate to fully leverage AI’s benefits. These challenges include issues related to cost, complexity, workforce implications, as well as ethical considerations, and data privacy concerns.
Cost
Put simply, the upfront cost of implementing AI technologies can be significant.
This includes expenses related to acquiring AI software or platforms, upgrading existing infrastructure to support new technologies, and procuring data storage solutions. Beyond the initial investment, ongoing costs include software updates, system maintenance, and potentially additional data processing and storage fees.
Complexity
Integrating AI technologies with existing legacy systems can be complex and technically challenging. Compatibility issues may arise, necessitating custom solutions or extensive system modifications. AI systems require high-quality, standardized data to function effectively. Ensuring data across various sources and systems is clean, consistent, and standardized poses a significant challenge for many organizations.
Workforce Implications
Adopting AI technologies often reveals a skills gap within the existing workforce. Employees may need training in data science, machine learning, or AI tool management, which can be time-consuming and costly. Integrating AI into existing operations requires significant change management efforts to address resistance among staff, restructure teams, and redefine roles and responsibilities. Ensuring a smooth transition and maintaining employee morale are critical challenges.
Ethical Considerations
AI systems can inadvertently perpetuate or amplify biases in their training data, leading to unfair outcomes or decisions. Addressing these biases and ensuring AI systems operate fairly and ethically is a significant challenge. Ensuring AI systems’ decisions are transparent and understandable is crucial, especially in critical applications. There’s also the challenge of determining accountability for decisions made by AI systems, particularly when those decisions have significant consequences.
Data Privacy
As AI systems process vast amounts of data, including potentially sensitive personal information, ensuring the privacy and security of this data is paramount. Companies must comply with data protection regulations such as GDPR in Europe or CCPA in California, which adds complexity to AI implementation.
Obtaining consent for data use and communicating how AI uses data are essential for maintaining trust with customers and partners. Ensuring ethical data use practices is a challenge that requires careful policy development and implementation.
Addressing these challenges requires careful planning, investment in training and infrastructure, ethical considerations, and a commitment to protecting data privacy.
Companies that successfully navigate these obstacles can harness AI’s power to drive innovation, efficiency, and competitive advantage in the automotive aftermarket and beyond.
AI Is Here To Stay
There’s no doubt about it: the automotive aftermarket industry is on the brink of an exciting transition into uncharted territory.
As brands continue to innovate their product lines to meet the changing demands of buyers, they must also consider shifting their operations to leverage tools like AI. It’s important to remember that AI is never going to be a replacement for human interaction or connection. Brands will still need to implement creativity, deliver world-class products, and foster a sense of community around their brands to be successful in this dynamic market.
PDM Automotive Is A Single Source of Truth In A Sea of Change
As your business grows and transitions in this new and exciting era, choosing the right partners to enact meaningful change across your organization is essential.
Our PIM platform allows aftermarket businesses to easily maintain product fitment and description data and efficiently distribute this data across multiple eCommerce platforms simultaneously. This allows aftermarket businesses to speed up product launches to outpace the competition, elevate the customer experience with detailed automotive product insights, and unify trading partner data through instant, powerful APIs with one source of truth.
Contact PDM Automotive today and schedule a demo to see how our platform will be instrumental as your business moves into this new era of change.
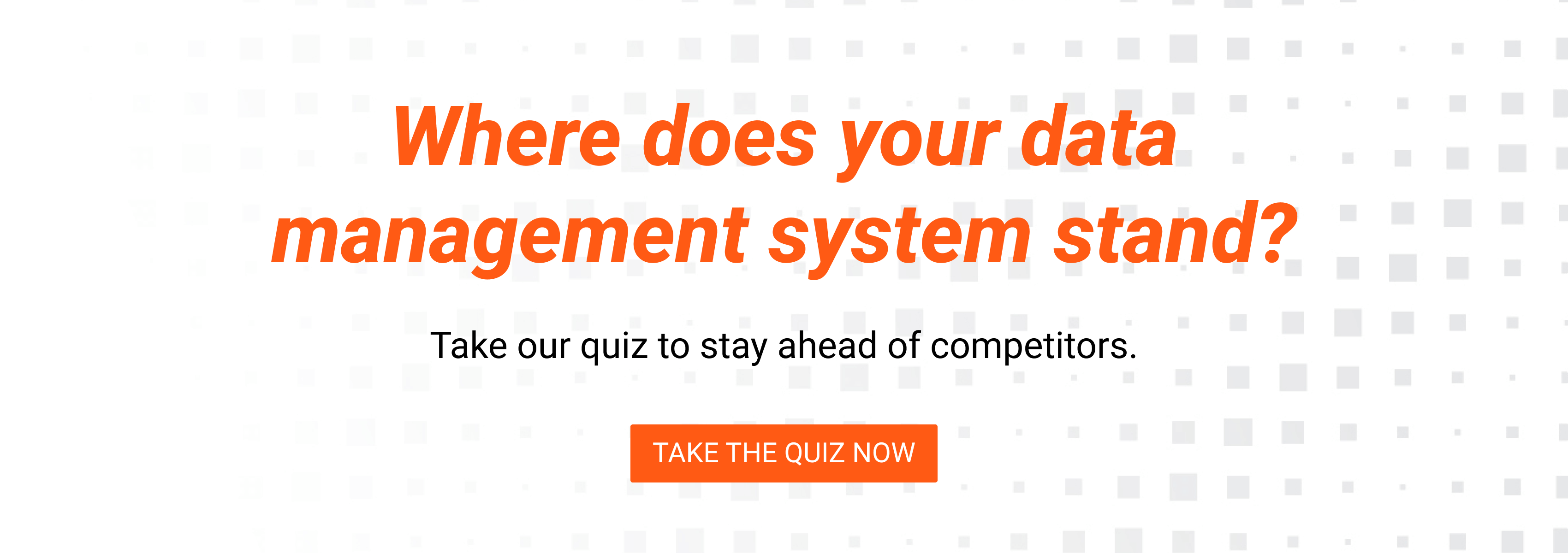
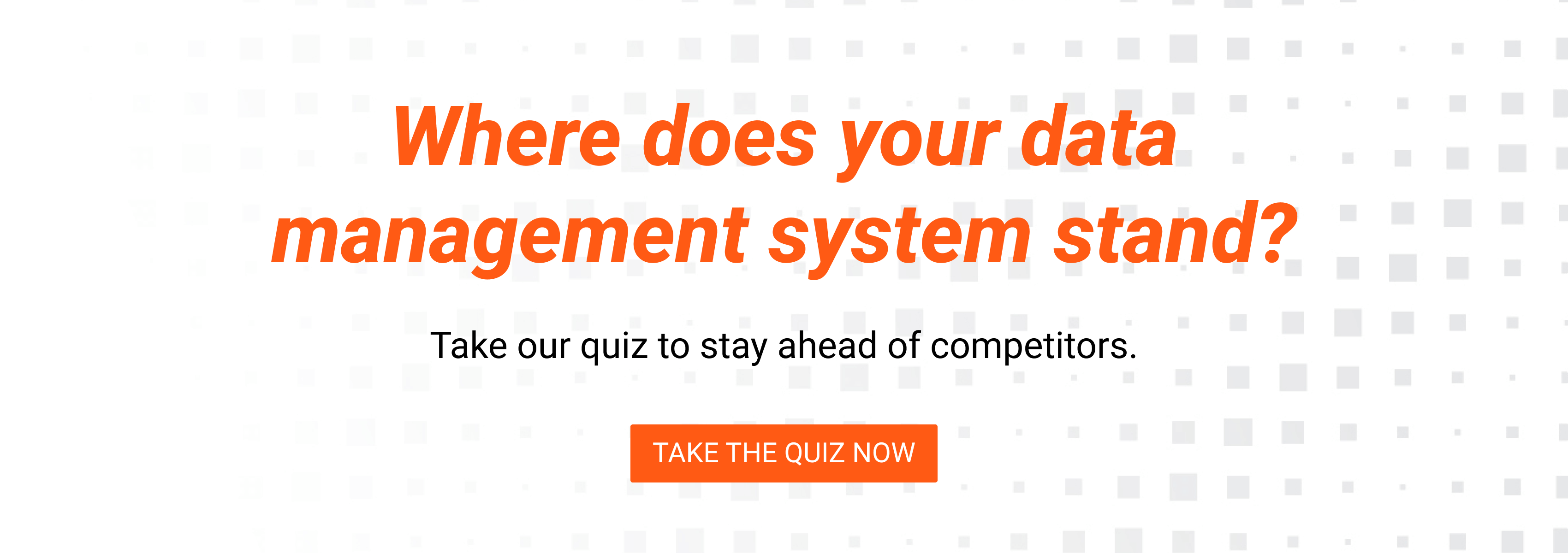
More Resources
Elevate Your Brand in the Automotive Aftermarket With These Content Management Strategies
A Guide to Creating High-Quality Web Content for Automotive Aftermarket Suppliers and Retailers